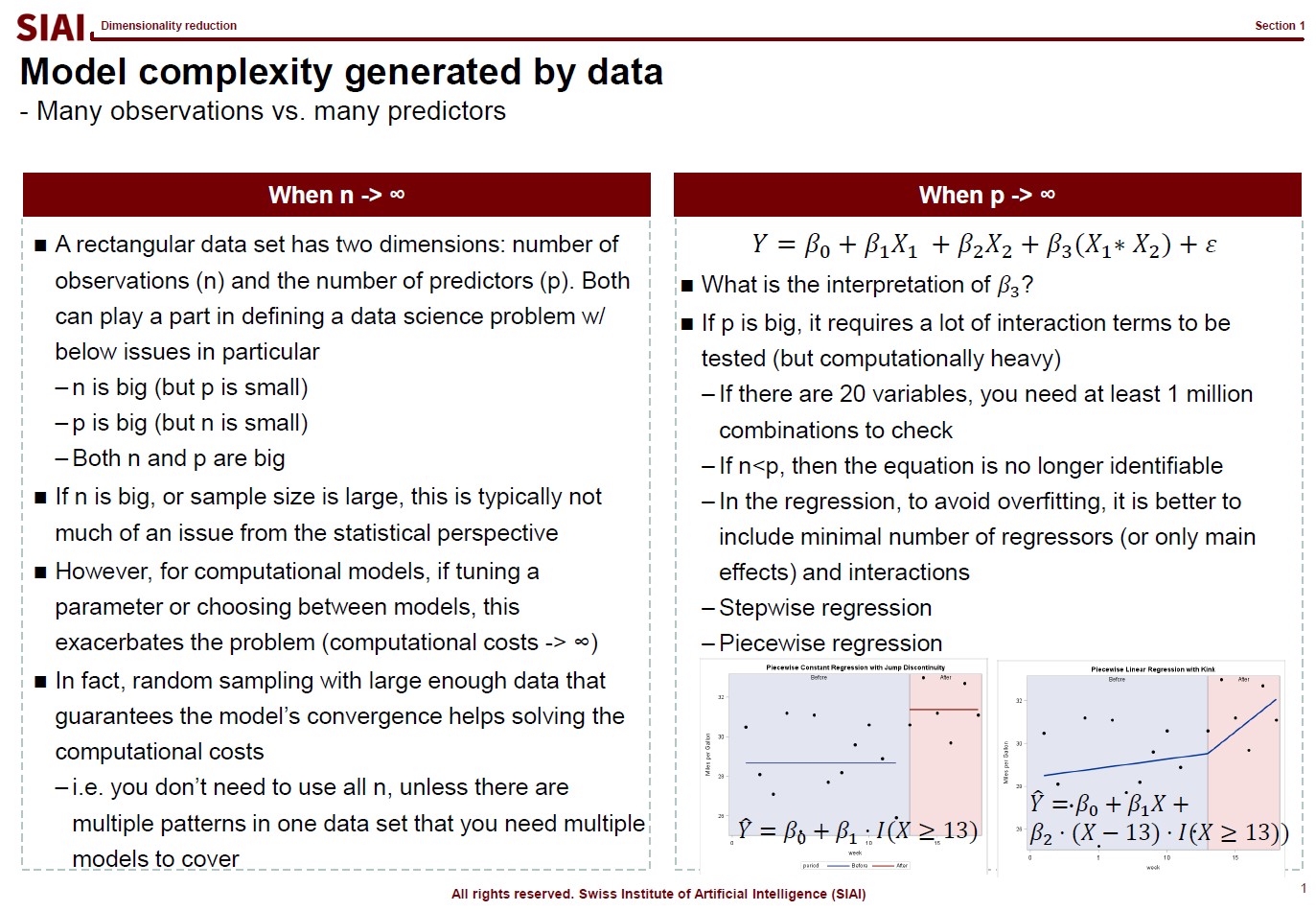
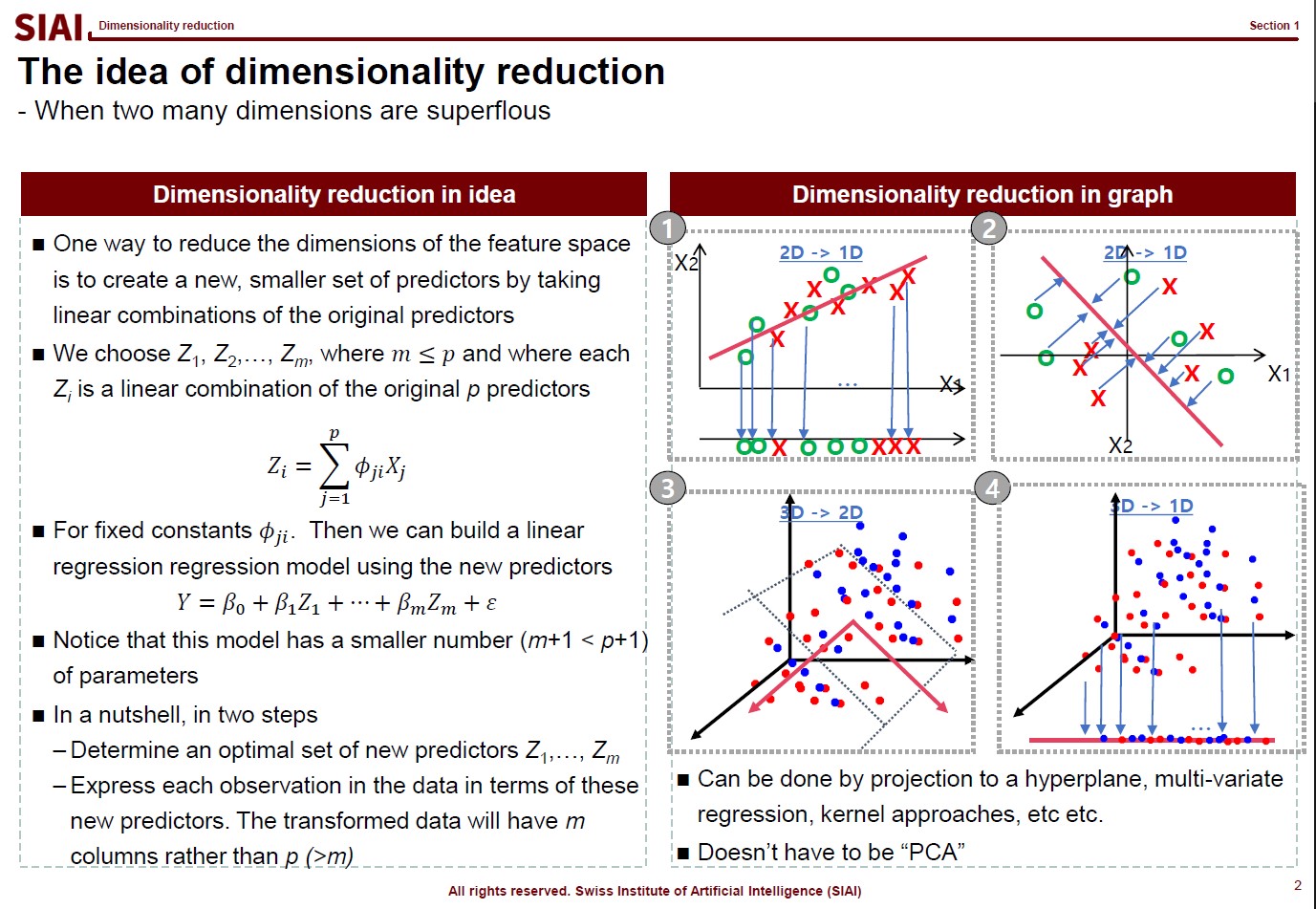
데이터 사이언스에서의 수학은 엄밀한 수학이 아니라 긴 문장을 짧게 표현한 것에 불과해데이터 사이언스는 수식이 의미하는 바를 직관적으로 이해하는 자세 필요해경제학에서 수학 기반 연구가 주류로 자리 잡은 이유는 수학이 효율적인 의사소통 수단이기 때문고등학교 때 수학이 가장 자신 있는 과목이자 가장 좋아하는 과목이었다. 당연히 대학교에 진학해도 수학을 좋아할 줄 알았지만, 대학 시절부터 수학은 싫어하는 과목으로 바뀌었다. 수학 성적이 박사 입학에 중요한 요소였기 때문에 수학 수업을 들었지만, 수년간 대학원에서 공부한 후에도 여전히 수학을 좋아하지 않는다(많은 사람들이 믿지 않지만). 단지 수수께끼를 풀 때 사용하는 수학을 좋아했다는 사실을 깨달았다.
People following AI hype are mostly completely misinformedAI/Data Science is still limited to statistical methodsHype can only attract ignoranceAs a professor of AI/Data Science, I from time to time receive emails from a bunch of hyped followers claiming what they call 'recent AI' can solve things that I have been pessimistic. They usually think 'recent AI' is close to 'Artificial General Intelligence', which means the program learns by itself and it is beyond human intelligence level.
Math in AI/Data Science is not really math, but a shortened version of English paragraph.
Korean GDP growth was 6.4%/y for 50 years until 2022, but down to 2.1%/y in 2020s.Due to low birthrate down to 0.7, population is expected to 1/2 in 30 years.Policy fails due to nationwide preference to leftwing agenda.